Data is the key to leveraging call center analytics - after all, how can someone make data-driven decisions without access to clean, organized data?
A call center stores lots of data, which is crucial to unlocking operational efficiency, overall productivity, and customer satisfaction. Decoding it manually can be highly complex and time-consuming.
As a contact center manager, team leader, VP or Quality Trainer, reviewing call center analytics is one of the most time-consuming tasks you will face.
Call center leaders can get overwhelmed navigating mountains of data to identify performance gaps, agent training needs, and areas for improvement to boost productivity.
Not only call center leaders but also agents are flooded with data from customer interactions, post-call data, and other sources such as the CRM.
.png)
Traditionally, making sense of the vast amount of call center data available involved manual analysis, consuming precious time and resources. With artificial intelligence (AI) involved in all day-to-day office activities, the processes have become much more sophisticated and mature.
Leveraging AI can automate analytics processes and provide actionable insights in real time, enabling swift decision-making and driving productivity.
We'll discuss call center analytics in detail, its relevance to call centers, the types of analytics, and how you can leverage AI to automate insights from your call center analytics data.
Jump to section:
- What are call center analytics
- Types of call center analytics data
- 5 common challenges of call center analytics
- How to analyze call center analytics
- How Generative AI can optimize your call center analytics data
- How to Amplify your call center analytics
What are Call Center Analytics?
Call center analytics is the result of gathering, analyzing, and interpreting data generated in a contact center environment. This analysis helps call center teams gain insights into various operations aspects, including agent performance, customer satisfaction, call volume trends, service level adherence, and more.
Call center analytics primarily aims to unveil actionable insights from agent interactions that drive better decision-making, deeper comprehension of customer needs, and improved business processes through data-driven problem-solving.
Contact center analytics leverage data from diverse sources and communication channels, including:
- Transcriptions of voice call
- Video recordings
- Chat histories
- Event logs
- Customer Relationship Management (CRM) software
- Interactive Voice Response (IVR) interactions
- Customer surveys
It is a common misconception that call center productivity metrics and KPIs are 'call center analytics' but the two are distinct and used for different purposes.
What's the difference between Call Center Analytics and Call Center Metrics (KPIs)?
The main difference between Call Center Analytics and Call Center Metrics (KPIs) is Call Center Analytics focus on data inputs whereas KPIs focuses on data outputs.
It's a common misconception that call center analytics are the same as key performance indicators (KPIs) and metrics, the truth is far more complex. Call center productivity metrics like average handle time (AHT), call volume, customer satisfaction scores, and average hold time are the results, or outputs, of deep analytical processes. These productivity and performance metrics are derived from analytics but do not encompass the analytics themselves.
Call center analytics sources are the backbone of getting accurate productivity metrics.
Types of Call Center Analytics
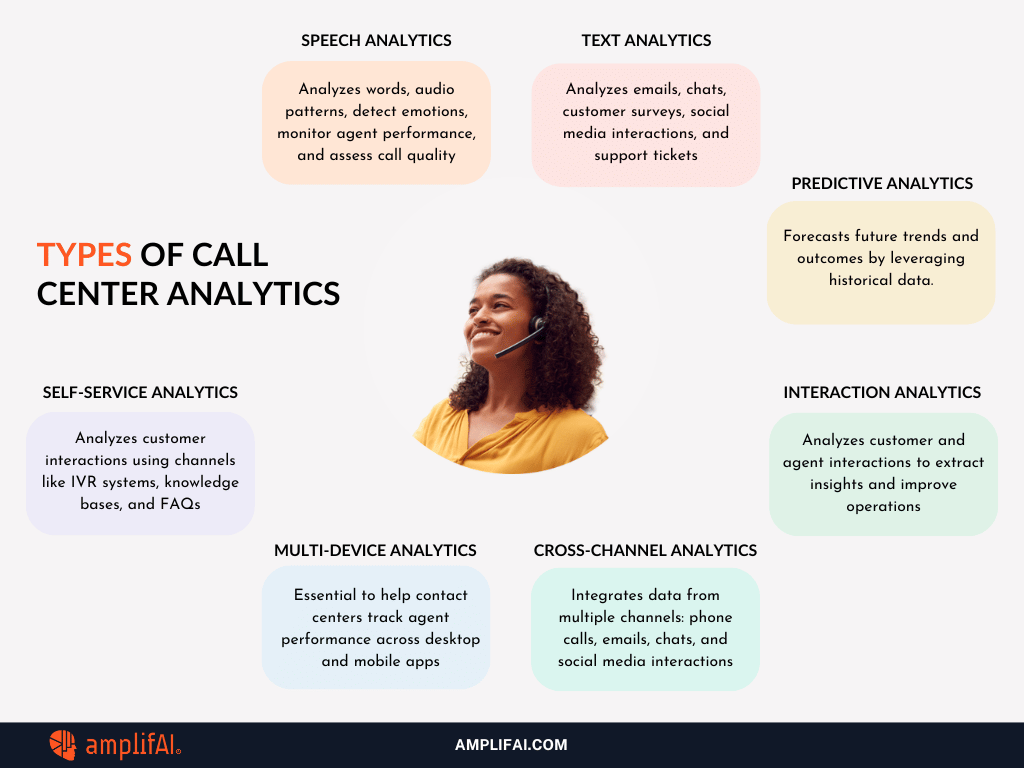
Given the amount of data available, call centers must determine which analytics will impact their operations and customer experience.
Each type of call center analytics data provides unique insights into different aspects of call center operations, customer interactions, and team performance. By leveraging these analytics effectively, you can improve efficiency, enhance customer satisfaction, and drive overall business success in your contact center.
The table below is the list of the top call center analytics:
Speech analytics involves leveraging AI to analyze recorded calls to extract valuable insights. It identifies words and analyzes audio patterns to detect emotions, monitor agent performance, and assess call quality.
According to McKinsey, speech analytics results in 20-30% cost savings and a 10% improvement in customer satisfaction scores.
Speech analytics comprises of:
- Identify keywords and phrases by scanning (specific positive and negative phrases or words) the customer interactions. This exercise will help you understand customer expectations, compliance issues, complaints, or missed service opportunities. It will also aid in categorizing interactions and identifying trends
- Sentiment analysis reveals the customer and agent's urgency, tone, and emotional state during the interaction. With this, you can identify if the customer is dissatisfied or satisfied during the call
- Categorizing calls based on topics or issues helps classify conversations into segments, which will provide insights into the most common customer issues, concerns, or complaints
With speech analytics, you can utilize the large number of conversations being stored and transform those into valuable insights that will benefit businesses by helping them discover trends, customer preferences, and opportunities for process improvement or training.
When analyzed correctly, speech analytics:
- Reduces customer effort by identifying pain points and improving self-service functionality
- Ensures quality assurance by monitoring conversations for script adherence and legal compliance
- Enhances agent coaching by identifying opportunities and providing real-time feedback
- It helps identify customer sentiment and ensures positive customer experiences
- Prompts agents to deliver relevant information, enhancing overall customer satisfaction
Like speech analytics, text analytics analyzes written communications such as emails, chats, customer surveys, social media interactions, and support tickets to extract valuable insights and improve call center operations.
Text analytics provide insights into customer preferences, common issues, and opportunities for improving written communication strategies.
Here's how text analytics works and its applications:
- Text analytics can determine the sentiment expressed in customer interactions (whether positive, negative, or neutral) to assess customer satisfaction levels and accordingly identify areas for improvement
- Categorize customer inquiries and issues into topics or themes, such as billing inquiries, product complaints, or technical support
- By analyzing text data over time, text analytics can identify emerging trends, recurring issues, or trends in consumer behavior, which can be used to address common problems and improve service quality proactively
- Text analytics can uncover the root causes of customer issues by analyzing the language and context used in customer interactions
- Assess agent performance by analyzing the language, tone, and effectiveness of their responses in written communications to identify training requirements and opportunities for improvement
- Analyze customer feedback collected through surveys, reviews, or social media to identify common themes, sentiment trends, and areas for improvement to enhance the customer experience
Predictive analytics helps you forecast future trends and outcomes by leveraging historical data. In call centers, predictive analytics can forecast call volumes, staffing requirements, customer behavior, and potential issues.
Contact center analytics uses AI to analyze call center data and discover future trends and patterns to address or ignore potential issues..
Call centers can proactively adjust staffing levels, resources, and processes by anticipating future trends to meet demand and improve efficiency.
6 Predictive Analytics Applications
- Predictive analytics will help forecast call volumes by analyzing past call volumes across different periods to better plan staffing levels and resources accordingly to meet demand.
- By analyzing historical call data, you can determine optimal staffing levels for different times of day, days of the week, or seasons, reducing overstaffing or understaffing situations.
- Analyze customer data and call patterns to anticipate customer needs, thereby allowing call centers to personalize interactions to enhance customer satisfaction and loyalty.
- Based on historical interactions, identify early warning signs of customer dissatisfaction. Call centers can then intervene with targeted retention strategies to prevent customer defection.
- Predictive analytics in contact centers will help boost agents' productivity. Monitor each interaction to discover reasons behind poor customer engagement or preempt similar issues customers face.
- Plan training programs for agents based on the lessons learned from their historical calls. Predictive analytics will help you understand agents' resolution time, the tone of their conversations, their inability to engage with specific customer issues, pronunciation mistakes, etc.
Predictive analytics empowers call centers to make data-driven decisions, anticipate customer needs, optimize operations, and enhance customer experience.
Call center interaction analytics analyzes customer and agent interactions to extract insights and improve operations.
Contact centers can use AI, machine learning, natural language processing, and neural networks to identify customer preferences and pain points.
Interaction analytics allows you to recognize recurring issues or dissatisfaction areas and work on improving overall customer satisfaction. It helps improve first call resolution (FCR) by identifying factors leading to repeated calls for the same issue, which enhances FCR rates.
Desktop and mobile analytics are essential to help contact center track performance.
Desktop analytics track the performance and movement of contact center agents using desktop computers. Meanwhile, mobile analytics monitors mobile devices, such as tablets and smartphones.
Together, desktop and mobile analytics can help you address slow bandwidth and security weaknesses. Teams can also monitor call center agents' apps and tools and whether they use them correctly.
They also help with real-time call monitoring by capturing inefficiencies, enhancing security, and identifying coaching opportunities for phone agents. This analysis can uncover redundant tasks, reduce call handling time, and improve overall efficiency, benefiting agents and customers.
Cross-channel analytics integrates data from multiple communication channels, such as phone calls, emails, chats, and social media interactions, giving a comprehensive view of customer calls and behavior across all touchpoints.
A call center must identify its customers' preferred channels and customize service accordingly. This helps them equip phone agents with this data, allowing personalized interactions.
For instance, if a customer primarily conducts banking online, agents can be alerted in real-time to offer online solutions. Just as with speech analytics, this area is emerging but likely to become indispensable in the future.
Cross-channel analytics help identify customer preferences, trends, and issues that span multiple channels, enabling call centers to deliver a seamless omnichannel experience.
Self-service analytics analyzes customer interactions using channels like IVR systems, knowledge bases, and FAQs.
Contact centers are now exploring methods to encourage self-service channels. For instance, if customers contact a call center agent to update their address, why not provide an online option on your website?
This helps minimize errors, decreases incoming call volumes, and reduces company costs.
Self-service analytics help optimize self-service channels, improve usability, and reduce call volumes by empowering customers to find answers and resolve issues independently.
5 Challenges of Call Center Analytics
.png)
Sometimes, too much of a good thing can become overwhelming, especially when it's not utilized effectively. This is the paradox of call center analytics: there's an abundance of data but often too little time and too few resources to make sense of it and turn it into actual and measurable performance indicators.
Inefficient use of data can hinder the effectiveness of call center operations and overall performance.
Below are 5 common challenges we see with call center analytics.
1. Making Sense of the Data
Call centers generate a massive amount of analytics data from various sources, such as calls, chats, emails, and customer interactions. Managing and making sense of this volume of data can be overwhelming for the managers as well as the call center agents.
2. Poor and Unstructured Data Quality
Ensuring the data's accuracy and consistency can be challenging, especially when handling data from multiple systems and channels. Poor and unstructured data quality leads to inaccurate insights and decision-making.
3. Data Stored in Siloed Systems
Data coming from different sources might be stored in siloed systems, making it difficult to integrate and analyze holistically.
4. Skill Gaps in Your Team
Not all call center staff may possess the necessary analytical skills to interpret and leverage analytics data effectively for decision-making. Effective utilization of call center analytics is essential for overcoming challenges, driving performance improvements, and delivering exceptional customer experiences.
5. The Drag of Manual Processes
Manual reporting processes are time-consuming and prone to errors. They limit the ability to analyze data in real time to make fast decisions. Traditional approaches to data analysis may need help scaling effectively to meet evolving needs as your call center operations grow in size and complexity.
Consequences of Poor Call Center Analytics Management
When the wealth of data available isn't harnessed correctly, the fallout extends far beyond inefficiency. Here are some of the most common—and preventable—consequences from poor call center analytics management:
1. Inefficient Resource Allocation
The lack of relevant data can lead to an inefficient allocation of resources, creating underutilization of staff or overburdening of agents, which can impact overall call center productivity.
2. Reactive Problem-Solving
Often, managers rely on intuition rather than data-backed facts and insights to resolve an issue, resulting in reactive problem-solving. In this case, problems are addressed only after they have escalated rather than being proactively identified and resolved.
3. Missed Opportunities for Improvement
Not effectively leveraging data means missing valuable insights that could drive operational efficiencies, improve agent performance, boost productivity, and enhance customer satisfaction.
By correctly leveraging the data, call center leaders can guide their agents in automating repetitive tasks, ultimately boosting productivity.
4. Stagnant Performance
Call centers that do not effectively utilize data find it difficult to adapt to evolving customer needs and market dynamics, leading to stagnant performance and loss of competitiveness. By leveraging data-driven insights, call centers can make informed decisions, and optimize operations.
.png)
Following the 8 steps below, you can analyze your call center analytics data to drive continuous improvement and deliver better customer experiences.
- Gather relevant data from various sources, such as call logs, customer surveys, and dynamics CRM services systems.
- Clean your data by removing duplicates, correcting errors, and standardizing formats.
- Identify key performance indicators (KPIs) such as call resolution time, average handle time, customer satisfaction scores, and first call resolution rate.
- Group data by relevant categories such as time of day, agent performance, and customer demographics.
- Visualize data using charts, graphs, and dashboards to visually represent trends, patterns, and correlations in the data.
- Analyze the data to uncover insights that can help improve call center operations, such as identifying common customer issues or opportunities for process improvement.
- Make data-driven decisions using the insights from the analysis to make informed decisions and implement strategies to improve call center performance and customer satisfaction.
- Effectively coach agents on the types of behavior associated with performance KPI improvement.
How Generative AI Can Optimize Your Call Center Analytics Data
We've established that managing the vast amounts of data and analytics generated daily in call centers can be overwhelming, often leading to missed opportunities and financial losses. But with the right application of Artificial Intelligence (AI), this flood of data can be transformed into a powerful asset, boosting overall call center productivity and enhancing service delivery.
Here are some examples of how contact centers leverage analytics and AI to optimize operations:
- Get actionable insights
- Predict call load and agent’s performance
- Enhance customer satisfaction
- Boost automation and efficiency
- Provide the right coaching opportunities
Using the power of Generative AI to manage and interpret call center analytics can move your team from data overload to strategic action.
While manually collecting data from various platforms — whether it's cloud-based, flat files, or APIs may offer a comprehensive view, integrating and actioning these disparate data sources poses a significant challenge.
Enter AmplifAI, a comprehensive call center performance management software designed to integrate and synthesize data from all your contact centers analytics sources in real-time.
From cloud CRM platforms, in-house QA tools to Excel files, AmplifAI integrates a diverse range of data sources across speech analytics, text analytics, predictive analytics, interaction analytics-all of your call center analytics.
By consolidating these data sources through AmplifAI's patented data ingestion process, Generative AI transforms complex datasets into clear, actionable insights, enabling your contact center leaders to focus on what matters— effectively coaching their frontline team.

AmplifAI eliminates the effort of managing and analyzing all reports and automatically consolidates and visualizes all the complex contact center data with ease to give insights that will help maximize agent productivity.
The platform is user-friendly and doesn’t depend on the IT teams. Once the data is ingested into AmplifAI a unified role based performance intelligence dashboard gives real-time strategies to improve the metrics that matter most to your contact center.
How AmplifAI Leverages Your Call Center Analytics Data
.png)
Integration Across Systems
AmplifAI connects with over 150 leading call center technologies, ensuring that data integration is smooth and comprehensive, spanning from cloud systems to on-premise applications. This integration is handled with minimal input from IT teams.
Unified Role Based Dashboard

Once your call center analytics data is integrated, AmplifAI's Generative AI and LLM interprets it to provide real-time insights, next best actions, and real time actionable recommendations.
Data-Driven Coaching and Performance Management

AmplifAI simplifies the process of effective call center coaching. By analyzing call center analytics across your team in real-time, AmplifAI identifies patterns creating high performer personas, using these insights to guide next-best-action strategies, coaching and development initiatives are tailored to boost the productivity metrics of all agents and team leaders in your contact center.
Agent Engagement and Recognition

AmplifAI uses your real time call center analytics data giving team leaders the gamification and recognition tools to motivate agents in real time.
Automated Quality Assurance & Compliance

AmplifAI's call center QA software automates the evaluation of agent interactions against QA benchmarks and goes a step further to tie this data into the specific and measurable performance metrics that matter to your contact center. This automation minimizes the need for tedious manual reviews and extracts valuable QA related information from your data.
Conclusion
In customer service, call center analytics is a vital strategic asset that impacts essential decisions (from operations to coaching).
By leveraging call center analytics with AI-backed technology, businesses can seamlessly convert customer interactions into opportunities for enhanced growth, operational efficiency, and exceptional service delivery.
Unlock the benefits of call center analytics with AmplifAI’s performance enablement platform. Book your demo now.